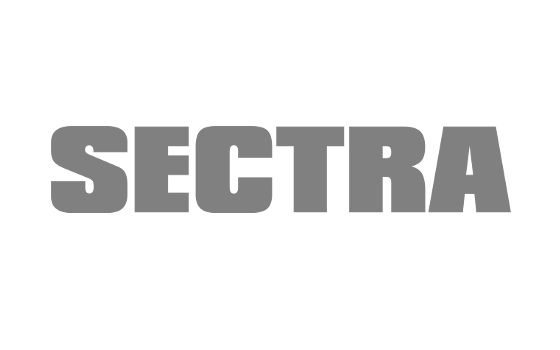
A new £21 million fund for AI was announced by the UK government in June, with the intention of providing NHS trusts with at least some of the tools needed to deliver faster, more accurate diagnoses for patients.
The urgency to get these tools in place, means that many NHS trusts and imaging networks will now be engaged in developing bids, ahead of what is a tight September deadline.
But what will those bids contain, and how can successful organisations start to make the most of funds? Here are five things some of those organisations bidding for funding might consider:
1. Have you identified the problem you need to solve?
With a significant focus expected on tools to help radiology teams as they report on many thousands of chest x-rays, NHS organisations do have the option to apply for funding for almost any AI application that supports diagnostics. But they also need to demonstrate value for money and a return on investment to be successful.Doing that starts with defining the problem that needs to be addressed. If healthcare professionals are not clear what an AI tool is trying to solve, it is unlikely to demonstrate improvements.
Clear goals need to be established that might mean improving patient outcomes or clinical efficiency, and that will also ideally have a monetary value associated.
For example, that might mean clearing a backlog of chest x-rays, reducing workloads for diagnostic teams under pressure, increasing the number of cases a radiologist is able to review, or improving turnaround times to support early diagnosis in particular areas.
2. How will you measure that?
To demonstrate value for money there needs to be something to measure before and after - particularly in relation to the problem you are trying to solve.Many algorithms will have compelling case studies. But you need to know if it works in your clinical environment, and for your population.
Every patient population is unique and there have been examples of AI working well in some places, whilst failing to work effectively for other patient cohorts or demographics.
Most organisations would choose a period of clinical validation. They might start with a retrospective study, and run it for a time period in test scenarios, whilst also creating milestones and KPIs to check and evaluate performance. If it works well, you can demonstrate the value to your organisation and others, and potentially scale deployment. If it doesn’t you might need to adjust your approach or try something new.
3. Can trusted evidence help to cut through the noise?
There is a rapidly growing number of AI vendors out there. New algorithms for healthcare seem to emerge almost every week. Any opportunities that can help your organisation to get a head start on what is likely to work, could help to deliver effective tools into clinical practice sooner.This can mean more than glancing at small scale case studies.
Strong peer-reviewed evidence of the efficacy of AI driven approaches to diagnostics, in some cases backed by substantial samples, is now being published.
One of our customers in Sweden recently made international headlines for a detailed research study involving more than 80,000 women, that showed significant potential for AI in helping to reduce workload for breast radiologists by as much as 44%.
Such peer reviewed studies are not likely to replace the need for local validation, but they can help to narrow the field from a large choice of proven and unproven tools.
4. What can you do to leverage regional resources?
The government's funding announcement focussed on trusts deploying AI tools. But that doesn't mean those trusts need to work in isolation.Imaging networks and regional consortia are continuing to mature in the NHS, and several of those regions we work with are electing to utilise their resources collaboratively.
For example, this could mean one trust out of five or six within a consortium, trialling an AI to detect lung cancers on chest x-rays, and sharing learnings with its partner trusts. Other trusts in the network might choose to trial other AI applications that perform a similar task, cutting down the time it takes to find the best tool for the job. Or they might trial AI applications in different areas and share what they learn.
5. Could your suppliers do some of the hard work for you?
NHS organisations will ultimately be responsible for investing the time needed to determine which AI tools are clinically effective for their patients.But a lot of time and energy could be saved in other areas. Many trusts for example lack the resource to manage commercial relationships with multiple suppliers. They might also not have enough bandwidth to manage the technical, data, integration and infrastructure complexities that can come with individual AI procurements.
The answer for many is to allow core system suppliers to carry some of this burden for them. In the case of imaging, trusts might wish to examine if their picture archiving and communication system (PACS) vendor can help to accelerate the deployment of AI, without the need for new contracts with additional suppliers.
Customers in the NHS using our Amplifier Service, for example, have told us that this approach has provided an ability to introduce AI easily and seamlessly into the radiology workflow, whist saving months of time and effort on IT and infrastructure work.